Text Analytics
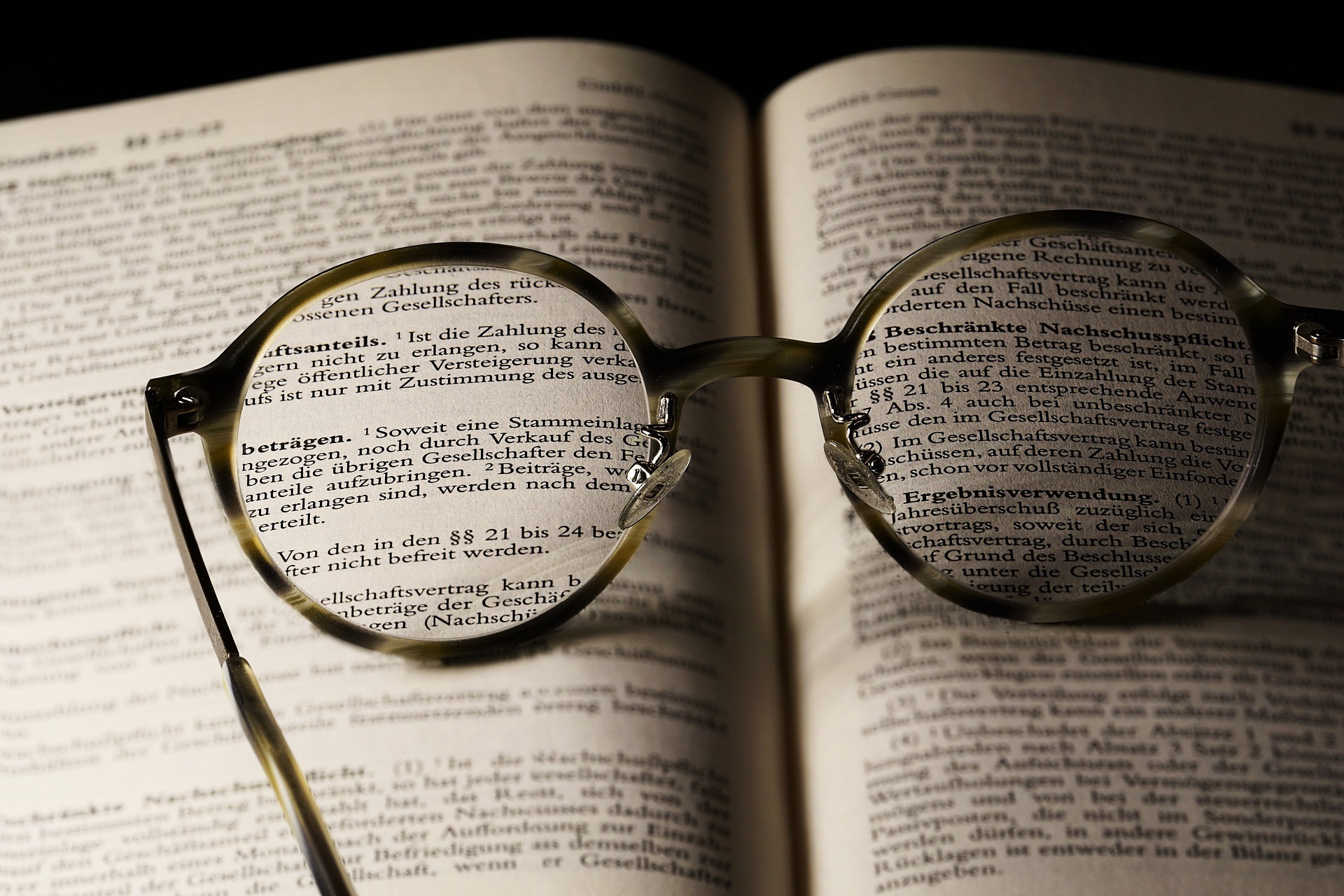
Text Analytics
Text analytics, a subfield of Language processing, is used to automatically extract and categorize useful information from unstructured text that is disseminated over the internet in the form of emails, tweets, conversations, tickets, ratings, and survey results. It makes it possible for organizations, governments, scholars, and the media to use the vast content at their disposal to make important decisions.
Among the methods used in text analytics include sentiment analysis, topic modeling, named entity identification, phrase frequency, and event extraction. There is a ton of content created every day in the forms of blogs, tweets, reviews, forum entries, and polls. Additionally, the majority of consumer contacts now take place digitally, which adds another massive text database.
Most text information is fragmented and disseminated across the internet. If the text data is gathered, assembled, appropriately arranged, and assessed, it can yield important information. Businesses can utilize these information to inform decisions that enhance revenue, customer satisfaction, research, and other areas.
Text analytics can benefit companies, groups, and even activist groups in a variety of ways. It supports companies in recognizing consumer trends, performance metrics, and service caliber. As a result, choices are made more quickly, business intelligence is better, productivity is increased, and expenses are reduced.
In a short period of time, researchers can examine a large body of prior literature and extract the data which is relevant to their research. It promotes quicker scientific advancements.
It helps in comprehending societal patterns and viewpoints, which in turn helps political entities like governments make decisions.
Search engines and information-gathering systems can perform better with the support of text analytics tools, leading to quicker user experiences.